Generative Deep Learning can Teaching Machines to Paint, Write, Compose, and Play
Generative Deep Learning has emerged as a revolutionary field with immense potential for transforming various creative disciplines. This article delves into the fascinating world of Generative Deep Learning and its ability to teach machines how to paint, write, compose, and play. By leveraging advanced algorithms and neural networks, Generative Deep Learning opens up new avenues for artificial intelligence to exhibit creativity and produce original artistic works. In this article, we explore the role of Generative Deep Learning in creative fields, uncover the techniques behind teaching machines to paint and compose, examine the intersection of Generative Deep Learning and music composition, discuss innovations in playing and performing, and consider the implications and future applications of this technology in the creative arts. Join us on this captivating journey into the realm where machines become artistic collaborators and creators.
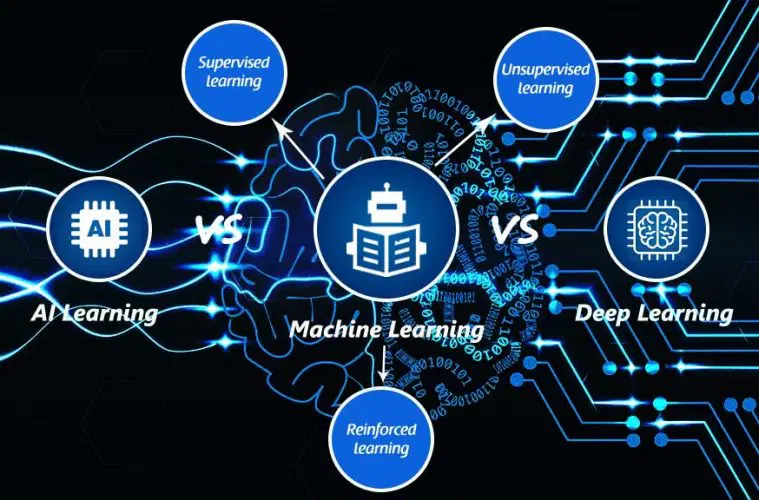
1. Introduction to Generative Deep Learning
1.1 What is Generative Deep Learning?
Generative Deep Learning is like having an AI-powered Picasso at your command. It’s a branch of artificial intelligence that focuses on teaching machines to be creative and produce original content, such as paintings, writing, music, and more. By using complex algorithms and neural networks, these machines can learn patterns and generate new, unique content that often surprises even their human creators.
1.2 Historical Background and Evolution
Generative Deep Learning isn’t just a passing trend; it has a fascinating history. It all began with the birth of neural networks in the 1940s, but it wasn’t until the breakthroughs in deep learning in the early 2010s that generative models really took off. Thanks to advancements in computing power and data availability, researchers were able to train deep neural networks to excel in creative tasks like never before. Today, generative models have become a hotbed of innovation, pushing the boundaries of what machines can do in the creative realm.

2. Understanding the Role of Generative Deep Learning in Creative Fields
2.1 The Relationship between Creativity and Deep Learning
Some people might argue that creativity is a purely human trait that machines can never possess. But generative deep learning challenges that notion by showing us just how creative machines can be. The algorithms used in generative models can discover and learn intricate patterns from vast amounts of data, enabling them to produce art, literature, and music that rivals human creations. It’s not about replacing human creativity, but rather collaborating and augmenting it with the power of AI.
2.2 Impact of Generative Deep Learning in Art and Literature
Generative Deep Learning has made quite a splash in the world of art and literature. Artists and writers are now turning to neural networks as their creative partners, using them to explore new artistic styles, generate novel storylines, and even create unique combinations of existing works. This technology has opened up exciting avenues for experimentation and has sparked a renaissance of creativity in fields that have traditionally relied solely on human imagination.
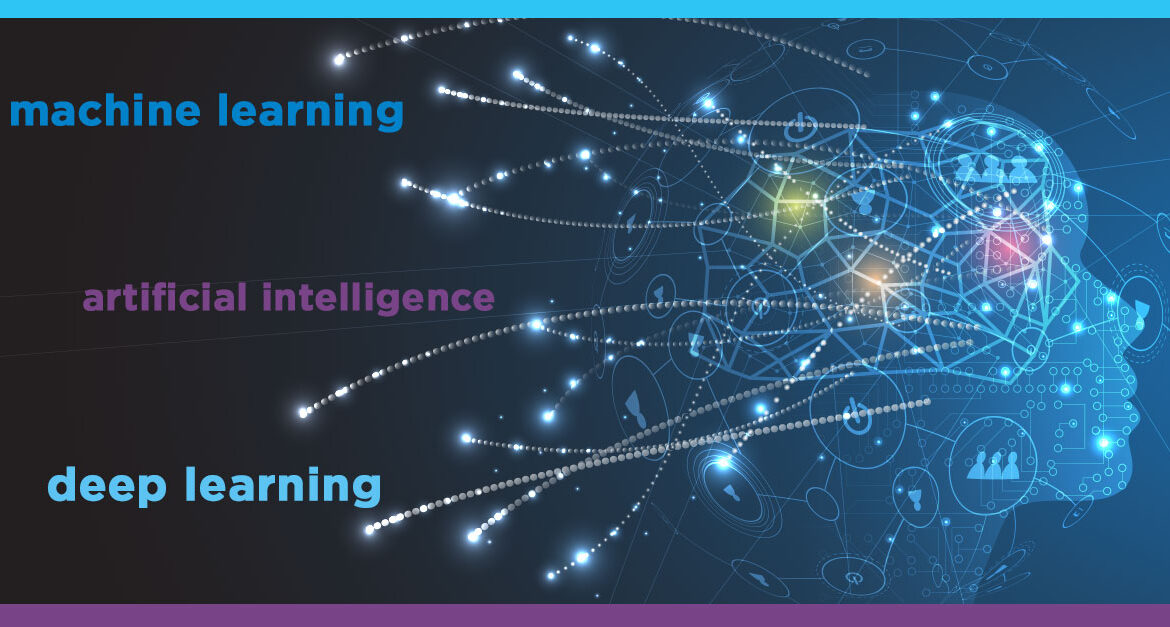
3. Teaching Machines to Paint: Exploring Deep Learning Algorithms
3.1 Overview of Painting and Deep Learning
Painting, meet deep learning. Deep learning algorithms have proven to be incredibly adept at understanding and replicating artistic styles. By analyzing vast collections of paintings, these algorithms can learn the underlying patterns and techniques used by various artists throughout history. This knowledge can then be harnessed to create stunning original artwork or even recreate the style of a particular artist with uncanny accuracy.
3.2 Generative Adversarial Networks (GANs) for Painting
One of the most popular techniques for teaching machines to paint is through the use of Generative Adversarial Networks (GANs). GANs consist of two neural networks: a generator and a discriminator. The generator creates new images, while the discriminator tries to discern whether those images are real or fake. Through continuous back-and-forth competition, the generator becomes better at producing realistic paintings, often surpassing our wildest expectations.
3.3 Style Transfer Techniques and Applications
Style transfer is another exciting area in painting with deep learning. By separating the content and style of an image, neural networks can recreate the content while applying the style of a different artwork. This technique has led to mesmerizing creations, allowing us to see famous landmarks painted by Van Gogh or a selfie transformed into a Picasso-esque masterpiece. Style transfer has unleashed the imagination of both artists and enthusiasts, making everyone an artist in their own right.
4. Writing and Composing: Harnessing the Power of Generative Models
4.1 Introduction to Writing and Composing with Deep Learning
Writing and music composition are quintessentially human forms of expression. But with generative models, machines can now participate in the creative process. Deep learning techniques, such as Recurrent Neural Networks (RNNs), have paved the way for machines to generate coherent and imaginative text. Similarly, these models can also compose musical pieces that captivate our ears and evoke a range of emotions.
4.2 Recurrent Neural Networks (RNNs) for Text Generation
Recurrent Neural Networks (RNNs) have become the go-to tool for teaching machines the art of writing. RNNs can process sequences of words and generate text that follows grammatical rules and has a coherent structure. They can even mimic the writing style of specific authors or generate entirely new pieces that showcase their own voice. With RNNs, machines have become aspiring authors, storytellers, and poets.
4.3 Music Generation with Deep Learning Techniques
The world of music has also been transformed by generative deep learning. By training neural networks on vast musical libraries, machines can now create melodies, harmonies, and even entire compositions. From classical symphonies to catchy pop tunes, AI-generated music has captured the attention of both music lovers and industry professionals. Who knows, the next chart-topping hit might just come from the creative circuits of a machine.

5. The Intersection of Generative Deep Learning and Music Composition
5.1 Generating Music with Deep Learning Models
Move over Beethoven, there’s a new composer in town, and it goes by the name of generative deep learning. This groundbreaking technology is making waves in the music industry by teaching machines to compose original pieces. Using deep learning models, algorithms are trained on vast amounts of musical data, enabling them to generate melodies, harmonies, and even entire compositions.
With generative deep learning, musicians and composers now have a new tool at their disposal. They can use these models to explore creative possibilities, find inspiration, or even collaborate with the machine. It’s like having an extra band member who never tires, never argues, and always comes up with fresh ideas.
5.2 Analyzing the Impact of Generative Music on the Industry
The introduction of generative deep learning in music composition has sparked a wide range of reactions within the industry. Some see it as a threat, fearing that machines might replace human musicians entirely. But let’s not get carried away just yet. Generative music is more like a helpful companion than a takeover plot.
Just as photography didn’t render painters obsolete, generative deep learning won’t wipe out human creativity in music. Instead, it brings new possibilities, challenges, and inspirations to the table. Musicians can use generative models as co-creators, aiding in the composition process. It’s a collaboration that encourages innovation and expands the boundaries of what is possible in music.
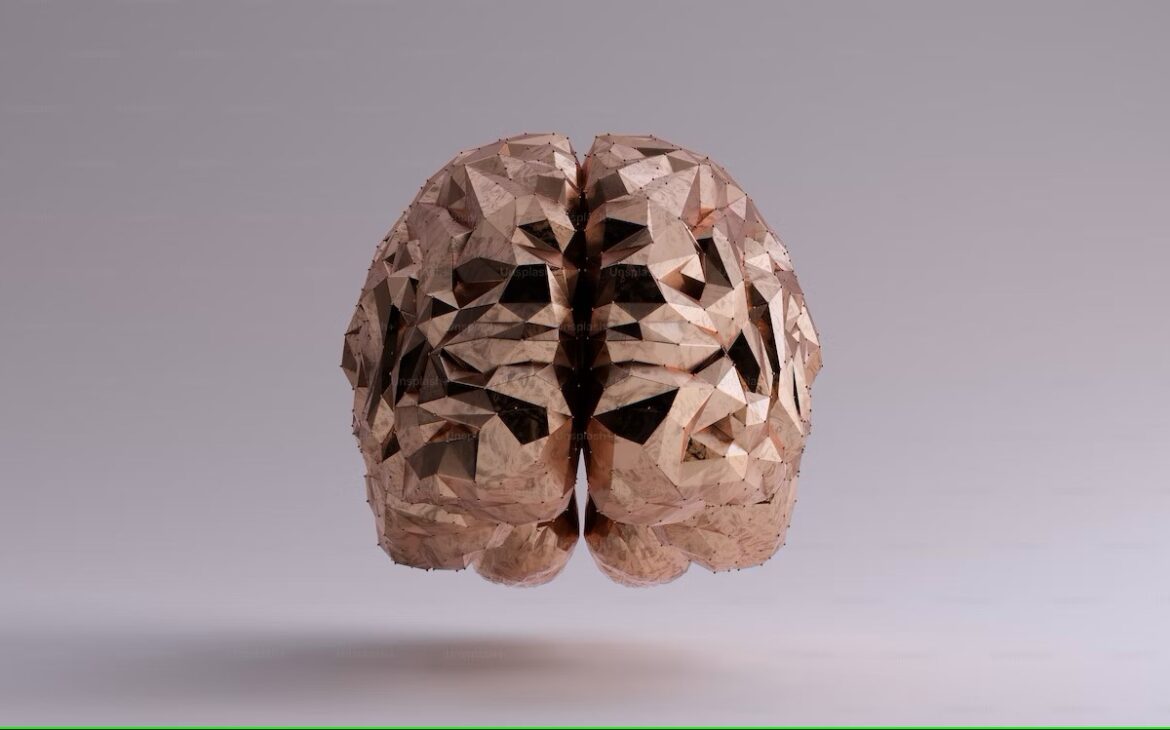
6. Playing and Performing: Innovations in Generative Deep Learning
6.1 Deep Learning and Virtual Instruments
Virtual instruments have been revolutionizing the music industry for years, and generative deep learning is taking them to the next level. By training models on massive datasets of instrument sounds, these algorithms can now simulate the behavior of real instruments and generate lifelike performances.
Musicians can now experiment with a vast array of virtual instruments, each with its own unique characteristics. Whether you want a symphony orchestra at your fingertips or a quirky collection of experimental sounds, generative deep learning can make it happen. It’s like having a virtual band at your command, ready to play whatever tune you desire.
6.2 Real-time Generation and Improvisation
Improvisation is a vital part of many musical genres, and generative deep learning models are now joining in on the fun. With real-time generation capabilities, these algorithms can respond to live performances and generate accompanying music on the fly.
Imagine jamming with a generative deep learning algorithm that listens to your playing and seamlessly improvises along with you. It’s like having a musical partner who can adapt to your every move, adding new layers of creativity and surprise to your performance.

7. Implications and Future Applications of Generative Deep Learning in the Creative Arts
7.1 Ethical Considerations in Generative Deep Learning
As generative deep learning continues to advance, it’s essential to address the ethical considerations surrounding its use in the creative arts. Questions arise about the ownership and originality of generative works. Does a machine-generated composition belong to the machine, the programmer, or the entity using it? These are complex issues that require careful examination to ensure fairness and proper attribution.
Additionally, there are concerns about the potential economic impact on human creators. While generative deep learning can open up new avenues for creativity, it’s crucial to strike a balance that ensures artists are still recognized and compensated for their work.
7.2 Future Directions and Potential Advancements
The future of generative deep learning in the creative arts is full of exciting possibilities. With ongoing advancements in technology, we can expect even more sophisticated algorithms that produce increasingly nuanced and expressive artistic outputs.
Imagine generative models that can capture and replicate the unique style of your favorite artist, or even create entirely new genres based on a fusion of existing ones. The potential for innovation and creative exploration is truly limitless.
As generative deep learning continues to evolve, it’s important to embrace its potential while staying mindful of the ethical considerations. By navigating this new frontier with care and creativity, we can unlock a world of artistic expression that was previously unimaginable. So let’s welcome our new generative companions and embark on this fantastic creative journey together.In conclusion, Generative Deep Learning has demonstrated its vast potential in teaching machines to paint, write, compose, and play. As this field continues to evolve, we can anticipate even more remarkable advancements in the intersection of artificial intelligence and creativity. With the ability to generate unique and compelling works of art, Generative Deep Learning is reshaping the boundaries of human imagination and artistic expression. As we navigate the ethical considerations and embrace the future applications of this technology, we must acknowledge the immense possibilities it holds for the creative arts. The journey of teaching machines to be creative collaborators has only just begun, and we eagerly anticipate the remarkable innovations that lie ahead.
FAQ
Q: What is Generative Deep Learning?
Generative Deep Learning refers to the use of advanced algorithms and neural networks to teach machines to generate original and creative content. It enables machines to autonomously create art, music, writing, and other forms of artistic expression, mimicking human creativity.
Q: How does Generative Deep Learning work?
Generative Deep Learning utilizes complex neural networks, such as Generative Adversarial Networks (GANs) and Recurrent Neural Networks (RNNs), to enable machines to learn patterns and generate new content based on those patterns. These networks are trained on vast amounts of data, allowing them to understand and replicate various artistic styles and techniques.
Q: What are some applications of Generative Deep Learning in the creative arts?
Generative Deep Learning has a wide range of applications in creative fields. It can be used to create original paintings, generate unique pieces of writing, compose music, and even simulate virtual instruments for playing and performing. These applications offer exciting possibilities for artists, musicians, writers, and other creative professionals.
Q: Are there any ethical considerations with Generative Deep Learning?
Yes, there are ethical considerations to be mindful of when using Generative Deep Learning. As machines generate content that can resemble the work of human artists, questions of ownership, copyright, and attribution arise. Additionally, the potential displacement of human artists or the devaluation of their work is a concern that needs to be addressed as this technology progresses.
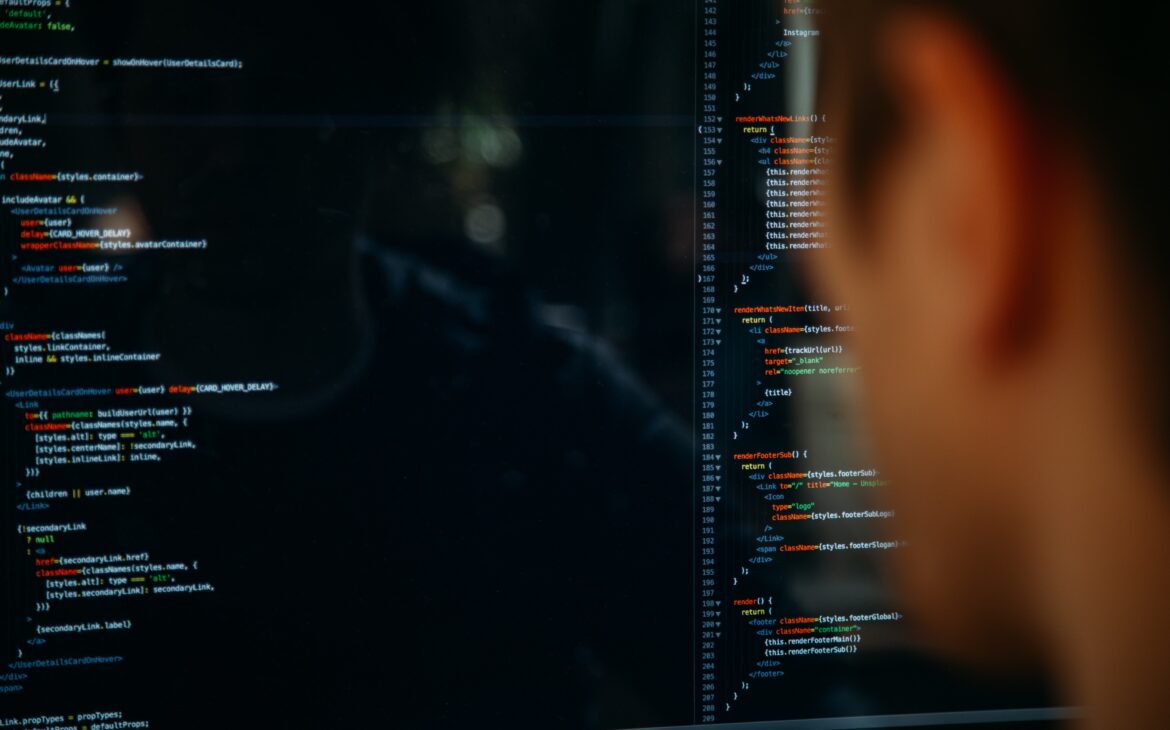
Conclusion
Generative Deep Learning, a subset of artificial intelligence, has shown immense potential in various creative domains. With its ability to learn patterns and generate new content, it can revolutionize the way machines engage in artistic endeavors.
Teaching machines to paint is one area where generative deep learning has made significant strides. By training on vast datasets of existing artwork, these algorithms can analyze brushstrokes, color palettes, and composition techniques. They then generate original paintings that mimic the style of renowned artists or create entirely new artistic expressions. This technology enables machines to produce visually stunning and thought-provoking pieces that blur the line between human and machine creativity.
Similarly, generative deep learning can teach machines to write compelling narratives or poetry. By analyzing extensive collections of literature across genres and time periods, these algorithms grasp the nuances of language, storytelling structures, and emotional arcs. They can then generate coherent stories or poems that evoke emotions and captivate readers. This capability opens up exciting possibilities for automated content creation in fields
If you want to build your website in an affordable price contact: www.nextr.in
Read this: A Beginners Guide To Understanding Artificial Intelligence