What is Liquid Neural Networks and How it Works?
In the ever-evolving world of artificial intelligence (AI), new frameworks and architectures are constantly being developed to the capabilities of machine learning models. One such architecture that has gained significant attention in recent years is the Liquid Neural (LiquidNN). This innovative approach to neural networks brings forth a unique way of processing and interpreting information, allowing for enhanced learning and decision-making abilities. In this blog post, we will delve into the world of Liquid Neural Networks, exploring what they are, how they work, and their potential applications.

Introduction to Liquid Neural Networks
Liquid Neural Networks (LiquidNN) is a type of recurrent neural network (RNN) that aims to simulate the complex dynamics found in the human brain’s cortical regions. By mimicking the behaviour of the brain, LiquidNN enables the efficient processing of spatiotemporal data such as speech, natural language, and temporal sequences of sensory information.
Unlike traditional feedforward neural networks that focus on processing input data sequentially, LiquidNN takes a different approach by leveraging the principles of liquid computing. This unique approach allows LiquidNN to process multiple streams of information simultaneously, leading to improved performance in tasks requiring pattern recognition, decision-making, and time-based predictions.
(If you are looking for Mobile Apps Development, Here you can click!)

How Liquid Neural Networks Work
To understand the inner workings of Liquid Neural Networks, it’s essential to grasp the concept of reservoir computing. Reservoir computing is a computational framework within which LiquidNN operates, enabling it to efficiently process and transform input data.
Reservoir Computing
Reservoir computing is a type of recurrent neural network architecture where the recurrent connections within the network form a “reservoir” of neurons. This reservoir acts as a dynamic memory, capturing and distilling the input data into a rich representation that can be readily interpreted and used for various tasks.
The reservoir is an untrained, fixed network of neurons through which input data propagates. This dynamic processing allows the reservoir to generate complex temporal dynamics that are inherent in the input data. The outputs of the reservoir neurons, known as the “reservoir states,” serve as a high-dimensional representation of the input data.
Training and Output Layer
In a Liquid Neural Network, the outputs of the reservoir states are linearly combined using a trainable output layer to generate the desired predictions or classifications. The output layer weights are updated through a training algorithm, such as ridge regression or support vector machines, which learns how to map the reservoir states to the desired outputs.
The key advantage of LiquidNN is that the reservoir, responsible for capturing and transforming the input data, remains fixed while only the output layer is trained. This separation of training the output layer from the reservoir allows for easier and more efficient training, as the complex temporal processing is already taken care of by the reservoir.
Get up to 70% Discount on Amazon (Buy Now)

Liquid Computing
Where the Liquid Neural Network truly distinguishes itself is through the concept of liquid computing. Liquid computing refers to the way LiquidNN processes information by simultaneously considering multiple possible interpretations or computations of the input data.
The reservoir acts as a dynamic system that takes advantage of the input data’s chaotic behaviour. As a result, the LiquidNN can handle time-varying inputs effectively, capturing their dynamics and allowing for robust and accurate predictions. This chaotic behaviour arises due to the random initial connections and nonlinear activation functions used within the reservoir.

Learning in Liquid Neural Networks
Training a Liquid Neural Network primarily involves two steps: the initialization of the reservoir and the training of the output layer.
During the reservoir initialization, the random connectivity patterns and activation functions are set. The values are selected or assigned randomly, ensuring a diverse range of representations within the reservoir. This diversity is vital for capturing the complex dynamics of the input data.
Once the reservoir is initialized, the output layer is trained to map the reservoir states to the desired outputs. Various training algorithms are employed to update the weights of the output layer, optimizing the mapping between the reservoir states and the desired outputs. This separation of reservoir and output layer training allows for fast adaptation to new tasks without modifying the reservoir.
(If you are looking for Mobile Apps Development, Here you can click!)

Applications and Advantages of Liquid Neural Networks
Liquid Neural Networks have shown great promise in a wide range of applications, benefiting from their ability to process spatiotemporal data efficiently. Here are some notable applications and advantages of LiquidNN:
1. Speech Recognition
LiquidNN demonstrates excellent performance in speech recognition due to its ability to process temporal sequences effectively. By leveraging the chaotic dynamics of the reservoir, LiquidNN can capture the subtle temporal changes in speech signals, leading to enhanced accuracy in recognizing spoken words and phonemes.
2. Time Series Prediction
The robust temporal processing capabilities of Liquid Neural Networks make them particularly well-suited for time series prediction tasks. Whether it’s financial forecasting, weather prediction, or analyzing physiological signals, LiquidNN can effectively capture long-term dependencies and complex patterns in the data, improving prediction accuracy.
3. Natural Language Processing
LiquidNN offers significant potential in natural language processing (NLP) tasks such as sentiment analysis, language generation, and entity recognition. The reservoir’s ability to capture the intricate dynamics of text data allows for improved context awareness and semantic understanding.
4. Robotics and Control Systems
Liquid Neural Networks find applications in robotics and control systems where time-varying and dynamic inputs need to be processed and interpreted. The ability of Liquid Neural Networks to handle real-time sensory information and complex temporal dynamics makes it valuable for controlling autonomous vehicles, robot arms, and other mechanical systems.
Get up to 70% Discount on Amazon Electronic Products

Advantages of Liquid Neural Networks
One of the primary advantages of Liquid Neural Networks is their ease of training compared to other recurrent neural network architectures such as Long Short-Term Memory (LSTM) networks. LiquidNN’s separation of reservoir and output layer training simplifies the training process, making it more efficient and less prone to overfitting.
LiquidNN also exhibits excellent generalization capabilities, allowing it to quickly adapt to new tasks without substantial modifications. The fixed reservoir provides a stable foundation for learning, eliminating the need for extensive retraining when new data or tasks are introduced.
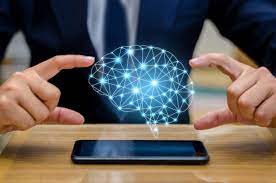
Conclusion
Liquid Neural Networks represent an exciting and innovative approach to neural network architectures. Their ability to leverage the principles of reservoir computing and liquid computing enables them to efficiently process and interpret spatiotemporal data for a wide range of applications.
With their applications in speech recognition, time series prediction, natural language processing, and robotics, LiquidNN holds significant promise for advancements in AI and machine learning. Its ease of training, adaptive capabilities, and robust temporal processing make it a valuable tool in various domains.
As the field of artificial intelligence continues to evolve, Liquid Neural Networks offer new possibilities for enhanced machine learning models. By incorporating the principles of the human brain into computational frameworks, LiquidNN opens the door to exciting advancements in AI and paves the way for future breakthroughs.
Thank you for reading 🙂
Get up to 70% Discount on Amazon Electronic Products

(If you are looking for Mobile Apps Development, Here you can click!)
Read the Next Blog Here: The Shocking Ways Technology Is Rewiring Your Brain